AI Personal Hotel Concierge for AYANA
Marvel Gomulya, CEO of Covena
|
March 28, 2025
|
11 min read

About 18 months ago, we started getting our first “AI agent” pitches. It was clear this had huge potential. But now we’re seeing the full map in even more clarity.
Quick a recap: We see AI Agents turning labor into software –– a market size in the trillions. Since our first essay on this, we’ve worked with amazing companies in this space and want to do more of it.
But if you’re following this space as closely as we are, you probably have noticed something. Progress and adoption are out of sync.
On one hand, there is rapid technological progress. Just recently, “tool use” (operator, CU, gemini 2.0) and improved “reasoning” (o3, R1, 3.7 Sonnet) emerged as new AI capabilities, both of which represent fundamental prerequisites for AI “agents”—and get us closer to the future. A world where AI Agents can act autonomously and execute complex tasks, at a far cheaper price than we thought possible even a few months ago is very real. Novel capabilities, paired with the continuous improvements in AI performance and cost (see Deepseek and this), are setting the foundation for future exploding demand. That’s the good news.
The less-good-news is there’s still a disconnect between progress and adoption. A gap between the intent to implement AI at work and actually doing it. For example, a recent McKinsey survey of 100 organizations doing >$50M AR recently found that 63 percent of leaders thought implementing AI was a high priority. But 91 percent of those respondents didn’t feel prepared to do so.
It’s very early days. And that’s where you come in. Your primary job is to be a bridge between deep technical progress and mass adoption. You have to figure out how to make people actually see this change, want it, and have it actually work for them.
So how do we get there? It turns out we may be missing a few layers of the AI agent stack.
Actually, we are missing three necessary layers right now, plus a bonus:
The Accountability Layer: the foundation of transparency, verifiable work and reasoning.
The Context Layer: a system to unlock company knowledge, culture, and goals.
The Coordination Layer: enabling agents to collaborate seamlessly with shared knowledge systems
Empowering AI Agents: equipping them with the tools and software to maximize their autonomy in the rising B2A (Business to Agent) sphere.
We are interested in companies building across each one of these layers. Or connecting them all, like NFX portfolio company Maisa (more on that below).


As we solve these challenges and build this infrastructure, we’ll be able to tackle new and more complex (and valuable) tasks with AI. And once that’s the norm, many more markets we can barely even conceive of now will emerge.
But first, we need these layers and here’s why:
Unlocking Autonomy: From RPA to APA (Agentic Process Automation)
To understand how we are going to unlock full autonomy, we first have to understand a major shift in the way people look at “process automation” –– for lack of a more interesting word.
We are moving from Robotic Process Automation (RPA) to an Agentic Process Automation (APA).
RPA is a multi-billion dollar industry with massive companies like UIPath, BluePrism, and Workfusion, among others. It’s proof of concept that people are more than willing to adopt automation for high value tasks. To understand how we can bring on the agent economy, it’s useful to use RPA as a starting point. Once you see its benefits and limitations, it’s clear how Agents are the natural, and massive, next step.
The benefits: RPA excels at rule-based, structured tasks spanning multiple business systems (100-200 steps). It was effective at capturing company knowledge within rules (e.g., VAT number processing), making automations reliable as long as underlying systems are static.
And, RPA has strong product market fit already.
The limitations: The universe of possible “RPA-able” tasks was always going to be limited because you had to, in detail, be able map out exactly what process the RPA should take. (Move a mouse here, design this spreadsheet that way, etc). And, importantly, expect it to remain the same. Or it breaks.
RPA can only go so far because you can’t process map, and expect perfect exact repeatability in everything you do (some companies can’t even process map at all without hiring outside consultants to “mine” their own processes). In fact, you may not even want that dynamic all the time – part of doing great work is reacting to an environment, intaking changes, tweaking things as you go.
In summary, RPA works extremely well for certain tasks. But RPA is completely inflexible. Reliable, but inflexible.
Enter LLMs — The rise of LLMs represents a major shift. LLMs provide unlimited, cheap, adaptive intelligence. They allowed us to define and collate the context needed to solve more complex problems. And as they began to learn reasoning, they hugely expanded the surface area of automatable tasks.
Marvel Gomulya
CEO of Covena
As Founders ourselves, we respect your time. That’s why we built BriefLink, a new software tool that minimizes the upfront time of getting the VC meeting. Simply tell us about your company in 9 easy questions, and you’ll hear from us if it’s a fit.
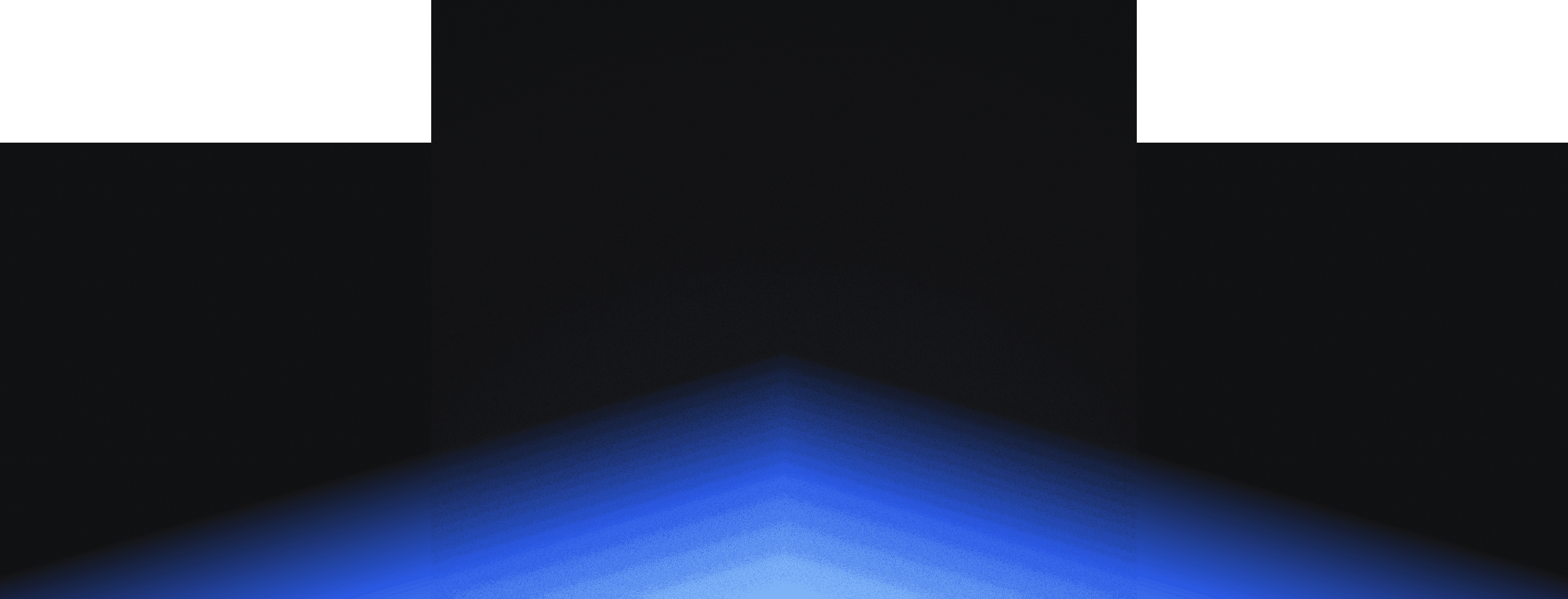
Ready to convert more customers with AI agents?
Covena
Human-like AI Sales Agents for B2C Companies, integrated directly to WhatsApp, Instagram, Email and more.
Terms of Service | Privacy Policy